Artificial intelligence is advancing astonishingly, and multimodal AI is one of its most exciting frontiers. Multimodal AI models, such as GPT-4 and Google Bard, transcend single-source inputs, combining diverse data types—text, images, audio, and sometimes even video—into unified, sophisticated systems.
For AI researchers, tech enthusiasts, and data scientists, multimodal models represent a monumental leap toward machines that think and respond more like humans. This blog explores multimodal AI, how it works, its current state, benefits, challenges, and where the future is headed.
What Are Multimodal AI Models?
Multimodal AI combines multiple data modes to create an integrated system capable of handling diverse types of inputs and generating cohesive outputs. Traditional AI models typically specialize in one modality, such as processing text (e.g., GPT) or analyzing images (e.g., Vision Transformers). Multimodal AI, however, bridges these modes.
For example, instead of merely analyzing an image or text, a multimodal AI model can, in a single query, examine a picture of a pie chart and explain its trends in natural language.
Why Multimodal AI Matters
The world operates in multiple modes of input. Humans don’t rely solely on text to comprehend things—we combine images, sounds, videos, and writing to understand concepts and solve problems. Multimodal AI uniquely mirrors this human-like reasoning, making it a game-changer in fields such as customer support, healthcare, robotics, and more.
The Current State of Multimodal AI
The Leaders in Multimodal AI
Several cutting-edge AI models are leading the charge in pushing multimodal boundaries.
- GPT-4 (OpenAI): The GPT-4 model is notable for integrating text and image analysis, enabling users to input documents containing pictures and receive context-rich responses. For example, it can analyze a scientific diagram and explain it concisely.
- Google Bard (Google): This experimental AI conversational model incorporates text and visual interaction, with a focus on enhanced reasoning and contextual understanding.
- DeepMind’s Flamingo: Known for its highly adaptable vision-language capabilities, Flamingo processes queries with image and textual inputs to provide insightful, real-time information.
- CLIP (OpenAI): A foundational model connecting language and vision for tasks such as generating captions or performing image classification with human-like accuracy.
Rapid Scaling
The above innovations show that enterprises are investing heavily in multimodal AI infrastructure. With platforms like these gaining more capabilities—further accelerated by AI tools that aid industries such as marketing and e-commerce—the momentum of multimodal AI is undeniable.
Advantages of Multimodal AI
1. Enhanced Understanding
Multimodal models facilitate deeper comprehension by processing combined inputs rather than siloed data. For instance, an AI model that analyzes both patient X-rays and accompanying diagnostic notes can make more precise assessments in a medical setting.
2. Boosted Creative Problem-Solving
By connecting multiple information streams, AI can draw parallels between disparate domains. For example, in autonomous vehicles, a multimodal AI system leverages cameras (visual modes), radar (audio modes), and text-based map inputs to make smarter decisions in real-world driving situations.
3. Improved Accessibility
Multimodal AI systems empower accessibility tools, such as live captioning for the hearing-impaired or picture-to-speech applications that help the visually impaired interpret their surroundings better.
4. Efficiency Across Fields
Whether enabling faster inventory tracking in retail by analyzing barcodes and labels, or enhancing chatbots with text and voice understanding, multimodal AI streamlines operations and opens doors for applications once considered science fiction.
Challenges and Limitations
1. Massive Data Requirements
Training multimodal models require immense datasets, often sourced from both structured (e.g., labeled captions) and unstructured formats. Gathering, cleaning, and aligning data can be complex, especially for rare modalities like medical imaging.
2. Computational Costs
These models demand significant computational power, making hardware requirements a prominent barrier. GPT-4, for example, requires millions of dollars worth of GPUs and energy inefficiencies for proper training.
3. Ethical Pitfalls
Multimodal models often exacerbate ethical concerns such as bias, misinformation generation, and misuse. For instance, when combining text and images, propaganda or deepfake content becomes harder to independently verify.
4. Context Understanding Still Has Gaps
At times, AI lacks appropriate reasoning when integrating multiple inputs. For example, multimodal models can still misinterpret sarcasm or fail to infer context from cultural idioms displayed in images.
Future Trends in Multimodal AI
1. Industry-Specific Applications
The future promises tailored multimodal AI solutions across industries.
- Healthcare: Models analyzing patient records and imaging will improve diagnosis accuracy.
- Education: Systems will enable real-time language coaching, combining speech-to-text inputs with interactive feedback tools.
- Entertainment: Personalization in gaming and movie-streaming will take a massive leap forward with multimodal cue detection, like eye movement scanned alongside preferences.
2. Self-Supervised Learning
One exciting avenue lies in self-supervised AI, where systems reduce data dependency through creative techniques that do not rely on labeled datasets.
3. Hardware Advancements
Custom-built chips optimized for efficient multimodal model computations will significantly reduce their energy costs and scalability issues.
4. Human-Centered AI
With developers focused on improving interpretability and bias reduction, the long-term vision for multimodal AI is centered on aligning its outcomes more closely with human ethical frameworks.
Why Multimodal AI is a Revolution
Greater than the sum of its parts, multimodal AI integrates multiple dimensions of data to provide deeper insights, better problem-solving, and scalable solutions. The field is set to redefine industries from logistics to law enforcement. Staying informed is necessary to ride this wave of transformation.
How You Can Keep Pace with Multimodal AI
This is just the beginning. Multimodal AI is reshaping the technological landscape, and businesses need to be ready. Whether you’re an AI researcher exploring possibilities or an industry leader considering practical applications, understanding and utilizing this technology could define the next phase of your success. Stay informed and watch this space for updates that could revolutionize how we interact with machines and data.
FAQs
What is the difference between multimodal AI and traditional AI?
Are multimodal AI models energy-efficient?
What industries will benefit most from multimodal AI?
Can I access tools like GPT-4 for multimodal queries?
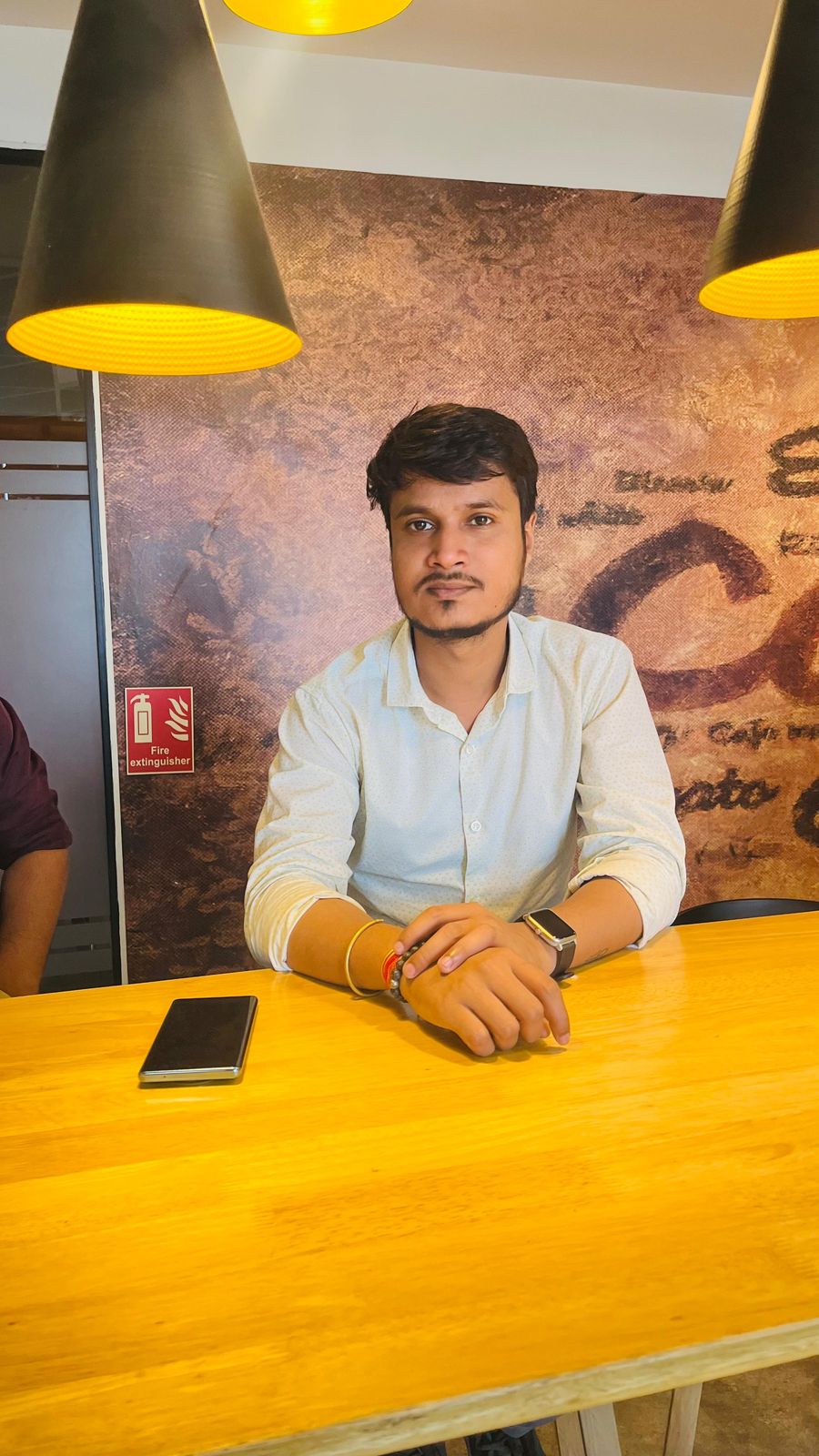
I am passionate about helping businesses grow their online presence and achieve measurable results. Let’s connect and discuss how I can help you reach your digital marketing goals!